Reinforcement Learning for Unknown Autonomous Systems
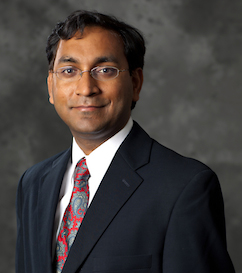
Speaker: Prof. Rahul Jain
Affiliation: University of Southern California
Abstract: System Identification followed by control synthesis has long been the dominant paradigm for control engineers, and yet, many autonomous systems must learn and control at the same time. Adaptive Control Theory has indeed been motivated by this need; but it has focused on asymptotic stability while many contemporary applications demand finite time (non-asymptotic) performance optimality. Results in stochastic adaptive control theory are sparse with any such algorithms being impractical for real-world implementation. We propose Reinforcement Learning algorithms inspired by recent developments in Online (bandit) Learning. Two settings will be considered: Markov decision processes (MDPs) and Linear stochastic systems. We will introduce a posterior-sampling based regret-minimization learning algorithm that optimally trades off exploration v. exploitation. This is a practical algorithm that obviates the need for expensive computation and achieves non-asymptotic regret optimality. I will then talk about a general non-parametric stochastic system model on continuous state spaces. Designing universal control algorithms (that work for any problem) for such settings (even with a known model) that are provably (approximately) optimal has long been a very challenging problem in both Stochastic Control and Reinforcement Learning. I will propose a simple algorithm that combines randomized function approximation in universal function approximation spaces with Empirical Q-Value Learning, which is not only universal but also approximately optimal with high probability.
Biography: Rahul Jain is the K. C. Dahlberg Early Career Chair and Associate Professor of Electrical Engineering, Computer Science* and ISE* (*by courtesy) at the University of Southern California (USC). He received a B.Tech from the IIT Kanpur, and an MA in Statistics and a PhD in EECS from the University of California, Berkeley. Prior to joining USC, he was at the IBM T J Watson Research Center, Yorktown Heights, NY. He has received numerous awards including the NSF CAREER award, the ONR Young Investigator award, an IBM Faculty award, the James H. Zumberge Faculty Research and Innovation Award, and is currently a US Fulbright Scholar. His interests span reinforcement learning, stochastic control, statistical learning, stochastic networks and game theory, and power systems and transportation on the applications side. The talk is based on joint work with a number of outstanding students and postdocs who are now faculty members at top places.
For more information, contact Prof. Suhas Diggavi (suhasdiggavi@ucla.edu)
Date/Time:
Date(s) - Jun 29, 2018
11:00 am - 12:30 pm
Location:
E-IV Tesla Room #53-125
420 Westwood Plaza - 5th Flr., Los Angeles CA 90095