Dealing with Perturbations in Compressed Sensing
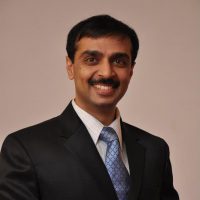
Speaker: Prof. Ajit Rajwade
Affiliation: IIT Bombay
Abstract: Compressed sensing (CS) is a sensing paradigm that proposes acquisition of images/signals directly in compressed format, thus reducing acquisition time. It has shown great results in speeding up MRI acquisition where time is critical, in improving frame rates of videos, and in general in improving acquisition rates in a variety of imaging modalities. In this talk, I shall present our group’s work on compressed sensing under noise in the measurement vectors or in the sensing matrix.
(1) In the first part, we consider the case where the compressive measurements are perturbed by noise that follows the Poisson distribution, which is the dominant noise model in optical or XRay imaging systems. This poses challenges in the theoretical analysis for CS, as the mean and the variance of the Poisson noise are both dependent on the unknown image. Most state of the art methods for “Poisson compressed sensing” have one or more of the following problems: (a) The error bounds have been developed for computationally intractable recovery algorithms, (b) The algorithms require knowledge of regularization parameters that are dependent on the unknown signal, (c) The analysis does not apply to “almost sparse” signals, and (d) The error bounds have not been developed for realistic models for the sensing matrix. We present two estimators for the underlying image which do not have these problems. Our work is easily extensible to the even more realistic scenario where the noise model is Poisson-Gaussian or Poisson-Gaussian-Uniform Quantization. The latter is a concrete practical challenge in this area, which has not been fully explored before.
(2) In the second part, we consider the case when the sensing matrix is perturbed, that is, inaccurately known. We focus on the specific case of the Fourier matrix, i.e. a system which measures the Fourier transform of image $x$ at some $m$ user-specified `base’ frequencies $u_1, u_2, …, u_m$. The measuring system may however be erratic and end up measuring the Fourier transform of $x$ at perturbed frequencies $u_1 + \delta_1, …, u_m + \delta_m$ where the values $\delta_1,…,\delta_m$ are bounded but unknown. This type of a situation is common in magnetic resonance imaging due to hardware errors, and also in a beautifully indirect way in tomography (the inner workings of a CT-scan machine). Ignoring these $\delta$ values during recovery of $x$ can lead to serious artifacts. We present a simple algorithm for this novel computational problem to compute the $\delta$ values along with the unknown $x$ and present some theoretical results related to it. We also discuss two other computational problem closely related to this, which crop up in radar imaging problems or multi-view compressed sensing. This is joint work with Karthik S. Gurumoorthy, ICTS-TIFR, and several masters and bachelors degree students from IIT Bombay.
Biography: Ajit Rajwade is an Assistant Professor in the Department of CSE at IIT Bombay. He obtained his PhD in 2010 from the Department of Computer and Information Sciences and Engineering at the University of Florida, Gainesville. He has a bachelor’s degree in computer engineering from the University of Pune, India, and a masters degree in computer science from McGill University, Montreal, Canada. He worked as a postdoctoral researcher in the Electrical and Computer Engineering Department at Duke University, Durham, North Carolina prior to joining IIT Bombay. His research interests are computer vision, and image and signal processing with a focus on compressed sensing, image restoration, tomography and inverse problems.
For more information, contact Prof. Achuta Kadambi (achuta@ee.ucla.edu)
Date/Time:
Date(s) - Nov 30, 2018
3:00 pm - 4:00 pm
Location:
E-IV Faraday Room #67-124
420 Westwood Plaza - 6th Flr., Los Angeles CA 90095