Next-Generation AI: An Integrated Software to Device Approach
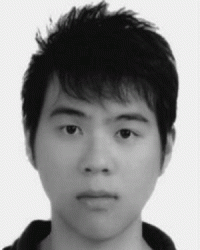
Speaker: Albert Lee
Affiliation: Ph.D. Candidate
Via Zoom: https://ucla.zoom.us/j/97349680908
Abstract: In recent years, artificial neural networks have contributed significantly to the advancement of machine learning, achieving state-of-the-art over a broad range of challenging tasks. The world right now is seeing a global artificial intelligence (AI) revolution involving academic and industry alike: commercial tech giants like Google and Microsoft are applying machine learning in their products, while professors from every discipline- software, engineering, mathematics, biology, transportation – scrambling to apply these methods to advance their research. Stock analysts are using AI to analyze and predict stock prices, medical experts to diagnosis and development of new drugs, and game developers for sophisticated, human-like decisions. At the national level, both NSF and DARPA have identified AI as one of the major national research directions.
Our research targets the advancement of next-generation AI from three vertically integrated aspects: At the software level, we propose the use of application-specific, bio-inspired models for audio and visual processing. We develop models of specialized neuron compatible with existing AI algorithms and optimize network structures to study their utilization. At the hardware level, we address the critical bottleneck in AI hardware: memory. We propose two schemes to improve performance and overcome limitations caused by variation of emerging devices. At the device level, we recognize the greatest computing boost comes from devices that compose AI computation via physical mechanisms. We propose two spintronic structures capable of computing convolutions, with orders of magnitude higher efficiency than state-of-the-art technology. These innovations provide the foundation for faster, more efficient AI at different temporal points along the next decade: in the short term, algorithms that can be implemented immediately; in the mid-term, hardware designs that can be realized in a few years; and in the long term, new device technologies to be adopted as the fabric of AI computation.
Biography: Albert Lee received the B.S. and M.S. degrees in electrical engineering from National Tsing Hua University, Hsinchu, Taiwan. He is currently working toward the Ph.D. degree at the Device Research Laboratory (DRL), University of California at Los Angeles, Los Angeles, CA, USA. His research interests include machine learning, nonvolatile logics/memory, and neuromorphic circuits.
For more information, contact Prof. Kang L. Wang (wang@ee.ucla.edu)
Date/Time:
Date(s) - May 27, 2021
2:30 pm - 4:30 pm
Location:
Via Zoom Only
No location, Los Angeles
Map Unavailable