Data Class-Specific Image Encryption Using Optical Diffraction
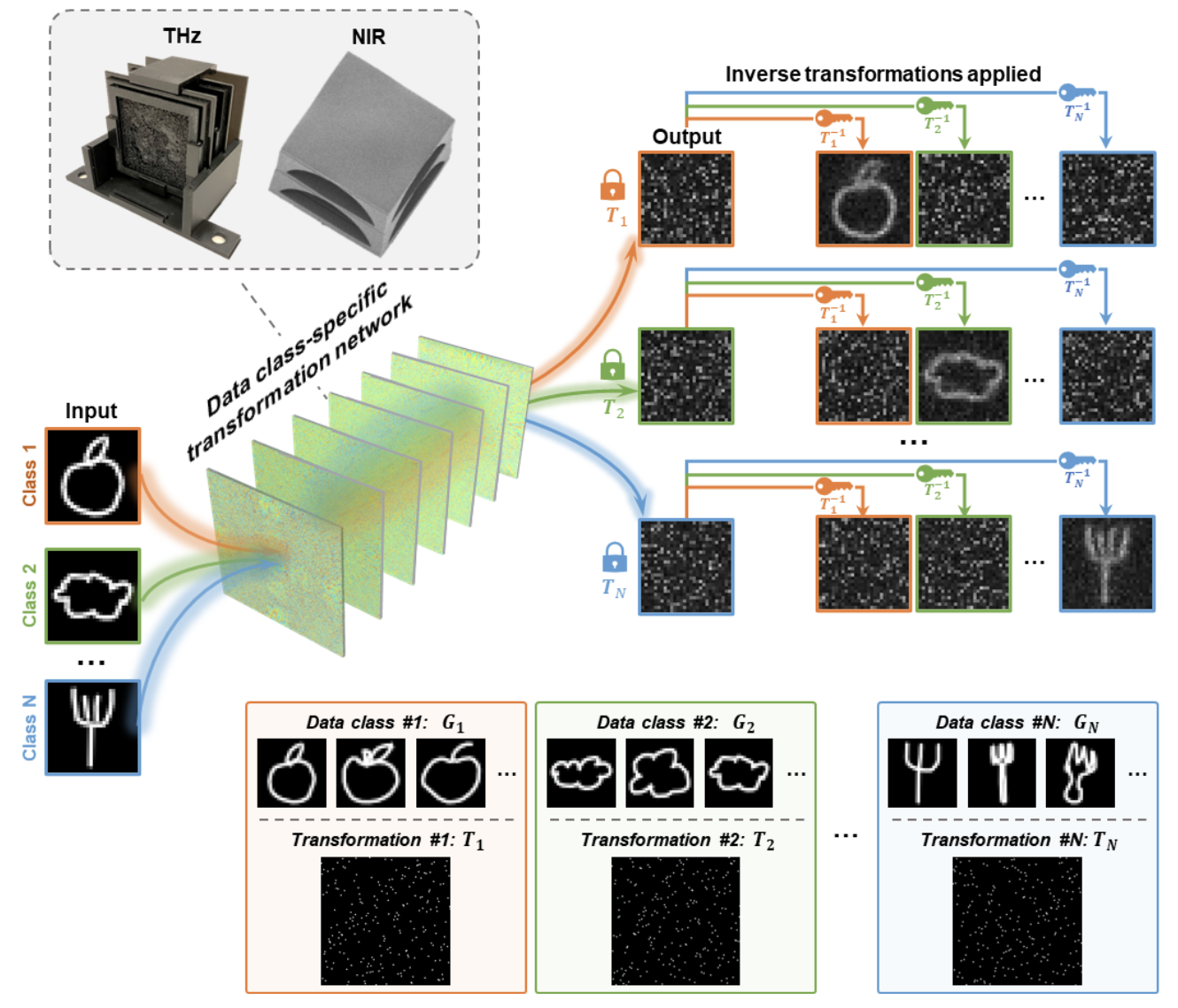
Optical computing has been making strides in recent years, with its potential advantages in speed, energy efficiency, and scalability. Among various photonic devices, diffractive deep neural networks (D2NNs) have gained growing attention as an emerging free-space platform for optical computing. D2NNs, also known as diffractive networks, use deep learning methods to design a series of spatially structured diffractive surfaces that modulate the light diffraction to compute a given task at the speed of light propagation. Besides its speed and energy efficiency, D2NNs also offer unique advantages for visual computing tasks, as they can directly process and access the 2D and 3D spatial information of a scene encoded by the amplitude, phase, polarization, and spectrum of the input light. This direct access to optical information makes diffractive networks ideal for visual computing tasks, such as image classification, hologram reconstruction, quantitative phase imaging, and seeing through random diffusers.
A team of researchers at UCLA has recently presented a diffractive network to perform data class-specific transformations and optical image encryption. In their paper, published in the journal Advanced Materials, UCLA researchers, led by Professor Aydogan Ozcan, demonstrated class-specific diffractive networks that perform the desired transformations for certain input data classes, resulting in optically encrypted images, which can only be recovered using the correct decryption keys.
In their results, the diffractive networks were trained using deep learning, and after their training was completed, they were physically fabricated using 3D printing to all- optically transform the input images and generate encrypted, uninterpretable output patterns captured by an image sensor. Only by applying the correct decryption keys (i.e., the class-specific inverse transformations) can the encrypted images be restored to reveal the original information, while applying other mismatched inverse transformations result in noise-like patterns. The UCLA team experimentally demonstrated the proof of concept of this class-specific all-optical image encryption at both near-infrared and terahertz wavelengths, validating its feasibility across different parts of the electromagnetic spectrum.
Instead of utilizing a fixed transformation matrix indiscriminately for all classes of input objects, this diffractive network-based image encryption scheme performs a set of pre-determined transformations, each specifically and exclusively assigned to one data class. In contrast, any other input images from undesired data classes will result in noninterpretable, meaningless output images. This class-specific encryption design adds an additional layer of security, and makes it more difficult to decipher the original images that belong to the target data classes by reverse engineering.
In addition to enhanced security, this class-specific design enables secure data distribution to multiple end-users, all simultaneously, using only one diffractive encryption network, where different decryption keys can be distributed to different receivers based on their data access permissions. This ensures that only the desired portion of the input data is shared with the authorized users, even though a single diffractive network optically encrypts all these different data classes.
This diffractive network-based data class-specific encryption is performed entirely based on light propagation through passive transmissive layers, requiring no external computing power other than the illumination light. This feature makes the system attractive for distributed image encryption using a fast, task-specific, and energy-efficient all-optical encryptor. This new diffractive image encryption design might pave the path to further the development of image and data security solutions and all-optical image processing devices operating at various illumination wavelengths.
This research was led by Dr. Aydogan Ozcan, Chancellor’s Professor and the Volgenau Chair for Engineering Innovation at UCLA, and an HHMI Professor with the Howard Hughes Medical Institute, in collaboration with Dr. Mona Jarrahi, UCLA’s Northrop Grumman Endowed Chair in electrical and computer engineering and Dr. Heming Wei, an Associate Professor at Shanghai University. The first author of this work is Bijie Bai, a graduate student from the Electrical and Computer Engineering Department at UCLA. The other authors include Xilin Yang, Tianyi Gan and Deniz Mengu, all from the UCLA Electrical and Computer Engineering department. Prof. Ozcan also has UCLA faculty appointments in the bioengineering and surgery departments and is an associate director of the California NanoSystems Institute.
The UCLA team acknowledges the support of the US Office of Naval Research.
See the article:
Bai, B., Wei, H., Yang, X., Gan, T., Mengu, D., Jarrahi, M. and Ozcan, A. (2023), Data Class-Specific All-Optical Transformations and Encryption. Adv. Mater. 2212091. https://doi.org/10.1002/adma.202212091