UCLA Researchers Develop Machine-Vision System Enabling Object Classification via Single-Pixel Detector
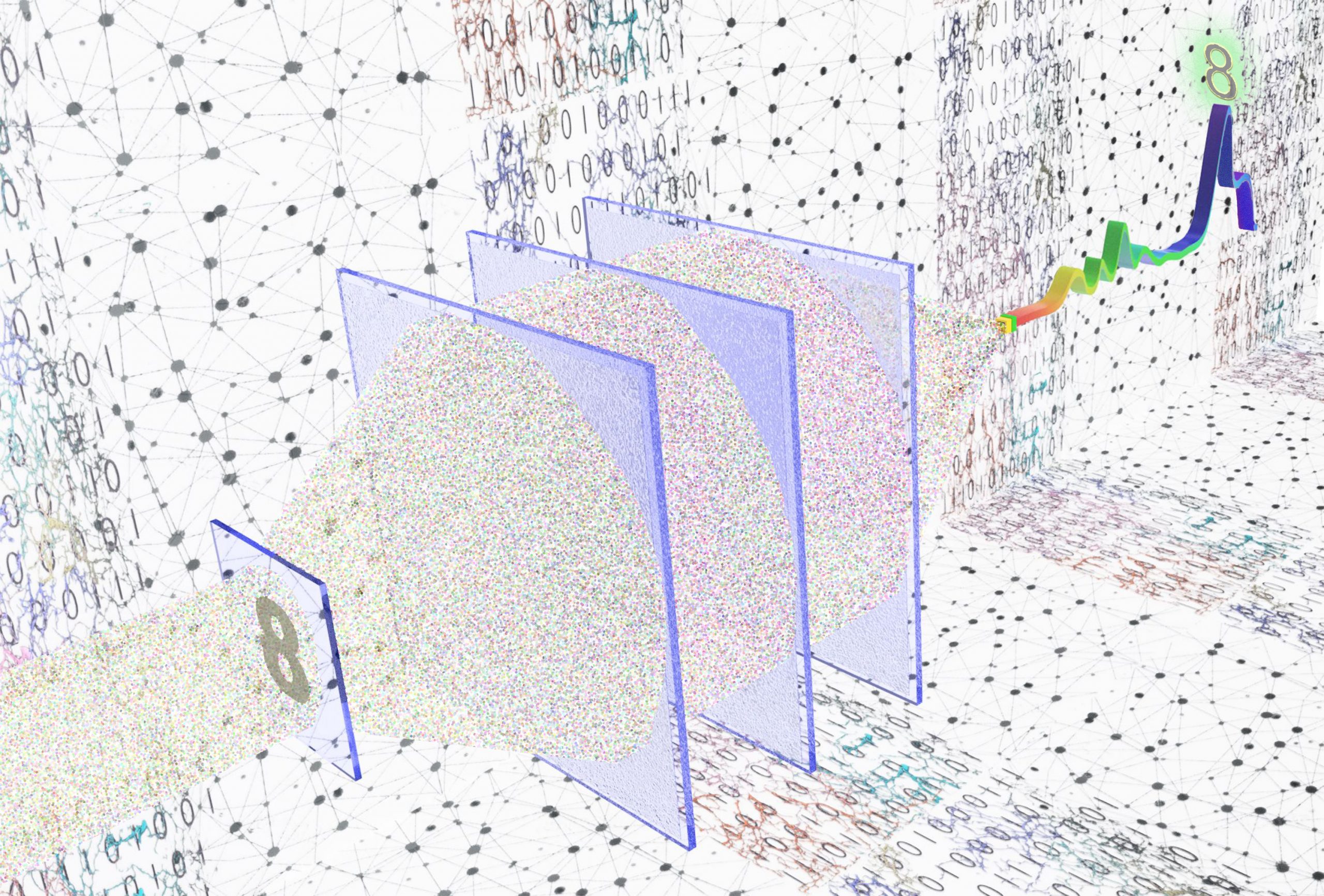
UCLA researchers created a single-pixel machine-vision system that can encode the spatial information of objects into the spectrum of light to optically classify input objects and reconstruct their images using a single-pixel detector in a single snapshot. Image credit: Ozcan Lab @ UCLA
Electrical and computer engineering researchers from the UCLA Samueli School of Engineering have developed a new machine-vision framework to overcome current drawbacks of handling and processing volumes of image data at high speeds. The advance could lead to enhanced machine-vision systems for self-driving cars, intelligent manufacturing, robotic surgery, biomedical imaging and other applications.
Instead of the traditional camera lens and computational systems, this new all-in-one model can classify the objects that it “sees” using a single-pixel detector in one snapshot.
The research was recently published in Science Advances, a journal of the American Association for the Advancement of Science. The project is led by Aydogan Ozcan, Volgenau Chair for Engineering Innovation at UCLA and the associate director of the California NanoSystems Institute, and Mona Jarrahi, director of the Terahertz Electronics Laboratory at UCLA. Both Ozcan and Jarrahi hold faculty appointments in the electrical and computer engineering department at UCLA Samueli.
Most current machine-vision systems involve lens-based cameras. After an image or video is captured, typically with a few megapixels per frame, a digital processor performs machine-learning tasks, such as object classification and scene segmentation.
According to the UCLA team, there are several drawbacks with this type of machine-vision architecture. First, the large amount of digital information captured makes it hard to achieve image/video analysis at high speed, especially when using mobile and battery-powered devices. In addition, these images usually contain redundant information, which overwhelms the digital processor, creating inefficiencies in terms of power and memory required. Moreover, fabricating high-pixel-count image sensors — similar to those found in mobile phone cameras — is challenging and expensive. These limitations also make standard machine vision methods harder to operate at longer wavelengths of light, such as at the infrared and terahertz parts of the spectrum.
The new, single-pixel, machine-vision framework is designed to mitigate such shortcomings and inefficiencies of traditional machine-vision systems. The researchers leveraged deep learning to design optical networks created by successive, diffractive surfaces to perform computation and statistical inference as the input light passes through specially designed 3D-fabricated layers.
“Unlike regular lens-based cameras, these diffractive optical networks process the incoming light at selected wavelengths in order to extract and encode the spatial features of an input object onto the spectrum of the diffracted light collected by a single-pixel detector,” said Ozcan. Based on this encoding, different object types or classes of data are assigned to different wavelengths of light. The input objects are then automatically classified, using the output spectrum detected by a single pixel, bypassing the need for an image sensor array or a digital processor.
“This all-optical inference and machine-vision capability through a single-pixel detector provides transformative advantages in terms of frame rate, memory requirement and power efficiency, which are especially important for mobile computing applications,” Jarrahi said.
The researchers demonstrated the success of their framework at terahertz wavelengths by classifying the images of handwritten digits using a single-pixel detector and 3D-printed diffractive layers. The optical classification of the input objects (handwritten digits) was performed based on the maximum signal among the 10 wavelengths that were, one by one, assigned to different handwritten digits (0 through 9).
Despite using a single-pixel detector, the team achieved an optical classification accuracy of more than 96%. An experimental proof-of-concept study with 3D-printed diffractive layers showed a similar finding with the numerical simulations, demonstrating the efficacy of the single-pixel, machine vision framework for building low-latency and resource-efficient machine-learning systems.
In addition to object classification, UCLA researchers also connected the same single pixel diffractive optical network with a simple, shallow electronic neural network. The process allowed the team to rapidly reconstruct the images of the input objects based on only the power detected at 10 distinct wavelengths, demonstrating task-specific image decompression.
The new framework can also be incorporated into various spectral domain-measurement systems, such as optical-coherence tomography and infrared spectroscopy, creating new 3D imaging and sensing modalities integrated with diffractive network-based encoding of spectral and spatial information.
The other authors on the study include UCLA Samueli electrical and computer engineering graduate students Jingxi Li, Deniz Mengu, Yi Luo, Xurong Li and Muhammed Veli, as well as post-doctoral senior researcher Nezih T. Yardimci and adjunct professor Yair Rivenson.